Demystify the Artificial Intelligence Words: List of 15 Essential AI Terms You Need to Know
As artificial intelligence (AI) continues to advance and become an essential part of our daily lives, it’s crucial to keep up with the ever-evolving terminology. In this post, I’ll walk you through the Artificial Intelligence words list, which will explore 15 essential AI terms, that you should know to better understand this fascinating field.
Importance of Knowing AI Terminology
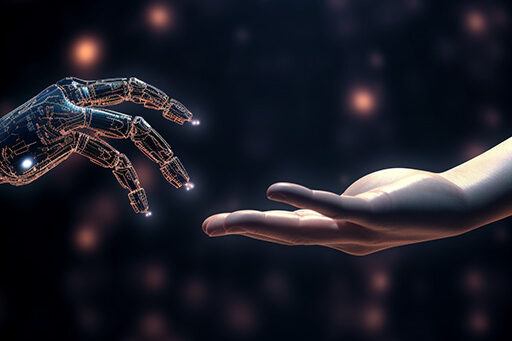
Before we explore the world of AI terminology, it’s essential to understand the importance of knowing these terms. As AI continues to make leaps and bounds in various industries like healthcare, finance, and transportation, it’s crucial to stay informed and educated about the technology driving these advancements.
Being familiar with AI terminology can help you make more informed decisions when it comes to buying new technology or software. Understanding how AI can benefit your business can save you tons of money and time. It will help you evaluate the claims made by AI-driven products and services. Additionally, knowing AI terms can help you engage in meaningful conversations with experts and contribute your thoughts on the subject.
Now that we’ve established the importance of knowing AI terminology let’s dive into our artificial intelligence words list – the top 15 AI terms you need to know.
Artificial Intelligence words list: 15 Essential Terms You Need to Know
1. Algorithms: the building blocks of artificial intelligence
An algorithm is a step-by-step procedure or set of rules for solving a problem or performing a task. In the context of artificial intelligence, algorithms are used to process data, learn from data, and make predictions or decisions.
2. Neural Networks
The neural network is a fascinating type of artificial intelligence that’s been modeled after the human brain! They are composed of interconnected artificial neurons that work together to process data and make decisions. Neural networks can be used to solve complex problems such as image recognition, natural language processing, and autonomous driving. Neural networks are often used in conjunction with other AI technologies such as deep learning and reinforcement learning to create powerful machine learning systems.
3. Machine Learning
Machine learning is a subfield of artificial intelligence (AI), that focuses on developing algorithms that allow computer systems to learn from data and make predictions or decisions without being explicitly programmed to do so. Machine learning involves training a computer program to recognize patterns in data, and then using those patterns to make predictions or take actions on new data.
In other words, machine learning is a way for computers to learn and improve by themselves without being specifically programmed.
4. Deep Learning
Machine learning and deep learning are related but not exactly the same. Deep learning is a subset of machine learning that involves using artificial neural networks to enable a computer to learn and make decisions in a way that resembles how the human brain works. Deep learning models can automatically discover and learn intricate features and patterns from large datasets, which makes them particularly useful for complex tasks like image and speech recognition. So, while all deep learning is machine learning, not all machine learning is deep learning.
5. Reinforcement Learning
Reinforcement learning is a type of machine learning where an agent learns to make decisions by interacting with its environment and receiving feedback in the form of rewards or penalties. The goal of reinforcement learning is to train the agent to maximize the cumulative rewards over time, enabling it to perform tasks such as playing games, controlling robots, and optimizing complex systems.
6. GANs – Generative Adversarial Networks
Generative Adversarial Networks (GANs) are a type of deep learning model that consists of two neural networks, a generator, and a discriminator, competing against each other. The generator creates fake data, while the discriminator evaluates the authenticity of both real and fake data. GANs can be used for tasks such as generating realistic images, enhancing low-resolution images, and creating 3D models from 2D images.
7. Natural Language Processing
Natural Language Processing (NLP) is a branch of artificial intelligence that deals with understanding and generating human language. NLP enables computers to understand and process written text, spoken word, and other forms of communication. It is used in a variety of applications such as chatbots, automated customer service agents, automated translation services, and document analysis. NLP techniques are also used to develop machine-learning models for tasks such as sentiment analysis and text classification.
8. Computer Vision
Computer Vision is a subfield of artificial intelligence that deals with processing digital images and videos to extract useful information. It uses techniques such as image recognition, object detection, facial recognition, and optical character recognition to interpret images and videos. Computer vision can be used in a wide range of applications such as autonomous driving vehicles, surveillance systems, medical image analysis, facial recognition systems, and robotics.
9. Robotics
Robotics is a field of AI that involves designing, creating, and operating robots that can perform tasks either autonomously or semi-autonomously. These machines are capable of working in hazardous or challenging environments, as well as taking on repetitive or boring tasks. The most exciting part is that AI plays a vital role in enabling robots to perceive their surroundings, make decisions, and even learn from their experiences. And did you know that robots can now do parkour and backflips? How cool is that!
10. Expert Systems
Expert systems are artificial intelligence programs designed to simulate the decision-making abilities of a human expert in a specific domain. These systems use a knowledge base of facts and rules, along with an inference engine, to draw conclusions and provide recommendations. Expert systems have been used in various fields, including medical diagnosis, financial planning, and natural resource management.
11. Data Mining
Data mining is the process of discovering patterns, relationships, and trends in large datasets using various techniques, including machine learning, statistics, and database systems. Data mining can be used for tasks such as customer segmentation, fraud detection, and market basket analysis.
12. Chatbots
Chatbots are AI-powered conversational agents that can interact with users through text or voice, providing assistance, answering questions, and performing tasks. Chatbots leverage natural language processing and machine learning techniques to understand user input, generate appropriate responses, and learn from user interactions.
13. Turing Test
The Turing Test, proposed by the British mathematician and computer scientist Alan Turing, is a test of a machine’s ability to exhibit human-like intelligence. In the test, a human judge engages in a conversation with both a human and a machine, without knowing which is which. If the judge cannot reliably distinguish between the human and the machine, the machine is considered to have passed the test.
14. Internet of Things (IoT): the future of connected devices
The Internet of Things (IoT) is the future of connected devices. It refers to the ever-growing network of physical objects that contain embedded technology to communicate and interact with their internal states or the external environment. IoT devices are connected to the internet, allowing users to control and monitor them remotely. These devices can range from smart home appliances such as thermostats and refrigerators to industrial machines such as robots and sensors. With the rise of 5G technology, we can expect even more powerful IoT solutions in the near future.
15. Blockchain
Blockchain is a distributed ledger technology that enables secure, immutable, and transparent transactions. It is most commonly associated with cryptocurrencies such as Bitcoin, but it can also be used for a variety of applications including smart contracts and digital voting. Blockchain technology allows data to be stored in multiple locations while still maintaining its integrity. By creating an immutable record of all transactions, blockchain ensures that data cannot be modified or tampered with without the consensus of all parties involved. This makes it an ideal technology for secure and transparent transactions.
Common Misconceptions about AI Terminology
There are several misconceptions surrounding AI terminology, often stemming from the use of terms interchangeably or the overhyping of certain concepts. For example, AI, machine learning, and deep learning are frequently used interchangeably, even though they represent different levels of granularity within the field. Another common misconception is the idea that AI and robotics are synonymous, while in reality, robotics is just one application of AI.
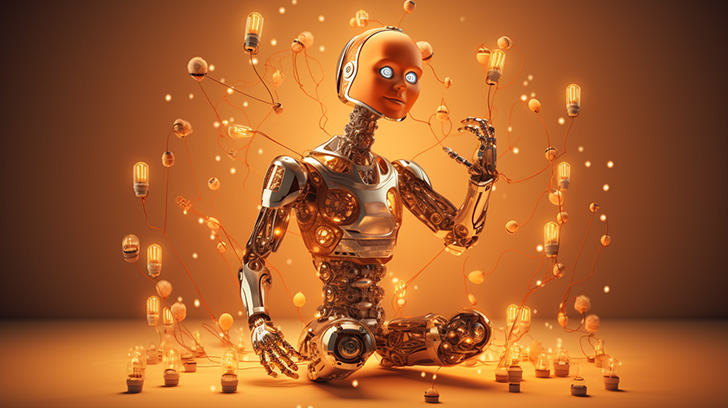
Another misconception is the belief that AI systems are inherently unbiased and objective. In reality, AI systems can perpetuate and even exacerbate existing biases in the data they are trained on, leading to unfair and discriminatory outcomes. Understanding these misconceptions is crucial for fostering a more accurate and nuanced understanding of AI and its potential impacts on society.
Conclusion
As AI continues to transform our world, staying informed about the key terminology is essential for anyone interested in understanding the technology and its applications. By familiarizing yourself with these 15 essential AI terms, you’ll be better equipped to engage in meaningful conversations about AI and make informed decisions about its use in your personal and professional life.
If you enjoyed this post and want to learn more about AI and other related topics, don’t forget to read my other blog posts!